AI embedding has emerged as a game-changer in the field of search. With its ability to transform the way we search for information, embedding holds the potential to revolutionise the search user experience. From search tools boasting about the volume of results returned to experiences where the user only sees the very best results, much closer to the interactions we have only seen up to know in science fiction. In this blog, we will explore the evolution of search from keyword-based systems to embedding, understand the limitations of keyword-based search, delve into the advent of AI in search, and explore real-world applications of AI embedding. Additionally, we will discuss the future of AI embedding, its impact on data privacy, and whether it can completely replace keyword-based search. So, let's dive deeper into the world of AI embedding and its game-changing potential.
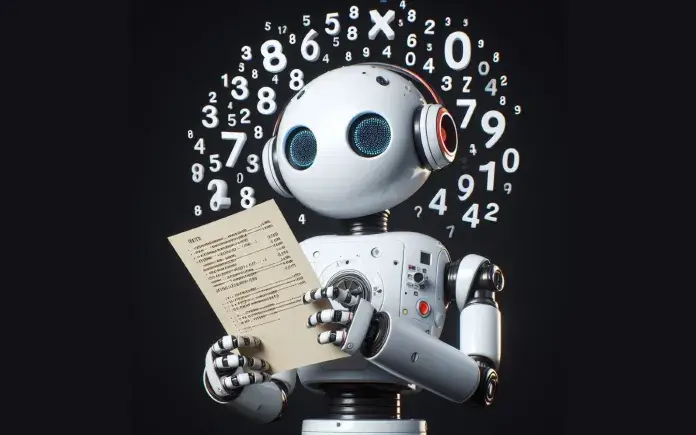
The Evolution of Search: From Keywords to Embedding
Over the years, the way we search for information has evolved significantly. It all started with keyword-based search, where users would enter specific terms or phrases to retrieve relevant information. This traditional search mechanism made use of predefined terms and phrases, matching them with user input to generate search results. However, as technology advanced, the limitations of keyword-based search became more evident, leading to the development of more advanced search mechanisms, including AI embedding.
What is Wrong with Keyword Based Search?
The limitations of keyword-based search became more apparent as users started searching for information using more natural language queries. Keyword-based search relies on exact matches of predefined terms, producing less relevant results when user queries don't align precisely with the predefined keywords. The inability to comprehend natural language and interpret user intent beyond specific terms hindered the search functionality.
The Advent of Artificial Intelligence and its Impact on Search Solutions
The advent of artificial intelligence has paved the way for more advanced search mechanisms and new business opportunities. With its generative capabilities, neural networks, and machine learning algorithms, it has revolutionised the search experience. By incorporating AI into search, developers have been able to enhance functionality and interpret user queries more effectively and hence more accurately find the right results. One such advancement is the concept of embedding, which has gained significant attention in recent years.
This has opened the door to entirely new business opportunities; companies like ConnectingYouNow have combined AI content ingestion with embedding and large language models to create conversational search tools that are optimised for non-technical users and those who would otherwise be excluded from participating in the Digital world.
AI Technology: What is Embedding and What it Means for Search?
In computer science, embedding refers to the process of representing data or objects in a lower-dimensional space. It allows for efficient and effective analysis and comparison of data, making it a crucial technique in various fields such as natural language processing and machine learning.
What this means for search is that each piece of content, be that text, image, video or audio, is converted, via an AI algorithm, into a vector with many hundreds of dimensions. What each of the dimensions relates to is controlled by the Embedding algorithm; they may, for example, relate to the number of legs of an animal in an image, the geographic location of an event referred to in an article or the maximum volume of a piece of audio.
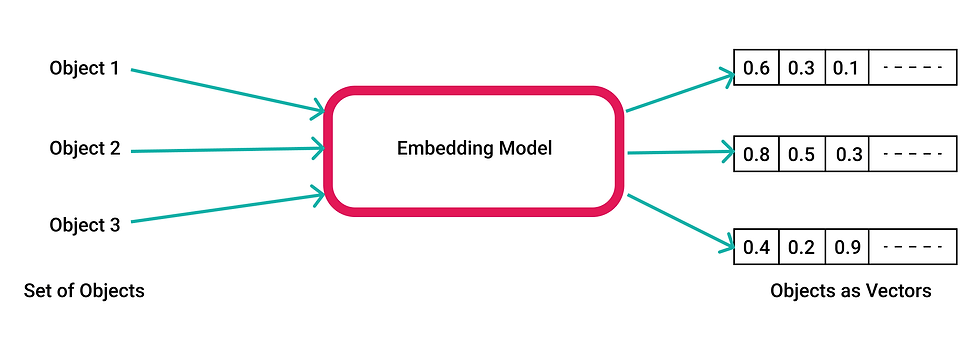
How Embedding Transforms Search Mechanisms
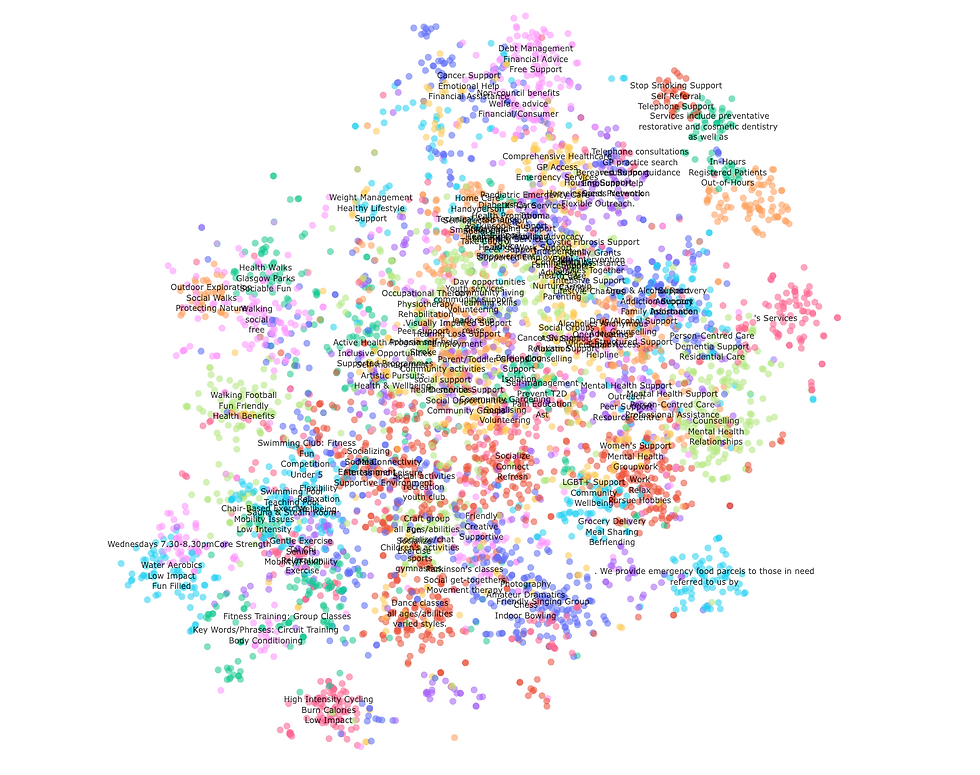
By converting information into vectors, AI models can better understand the similarities, differences, and relationships between words, phrases, and documents. Rather than performing exact matches the AI algorithms perform nearest neighbour searches, aiming to identify the results that are closest to the users request. This enables search engines to provide more accurate and relevant search results, ultimately improving user satisfaction.
Vectors are a series of numbers and numbers are what computers are most adept at handling. This means that large amounts of data and ever increasing amounts of new data can be efficiently stored and compared.
AI transforms search mechanisms by harnessing the power of ai algorithms, generative AI, neural networks, and machine learning algorithms. These technologies work together to analyse user queries, understand their intent, and deliver more accurate search results. Incorporating AI in search allows for more advanced AI-driven functionality, enabling search engines to go beyond exact matches of predefined keywords and provide more relevant information to users.
All the time people match similar terms to help them with problem solving, knowing that cyan is closely related to blue and in turn deep blue, for example is something people just apply, embedding allows AI to more closely match human intelligence in this way.
The Role of Vector Databases in AI Search
Vector databases are part of the AI tools arsenal that are optimised specifically and primarily to store and rapidly retrieve data stored as vectors. Where a traditional database uses a very few values as keys that allow rapid information retrieval, a vector database uses the whole vector. A traditional database is optimised to retrieve on exact matches, Vector databases are optimised to retrieve on proximity - the nearest matches are returned.
Vector databases play a crucial role in enhancing the latency and search functionality of AI systems. By storing precomputed vectors of information, search engines can perform real-time searches, reducing the time it takes to retrieve results. This real-time capability significantly improves the user experience, enabling faster access to relevant information. With the integration of vector databases, AI search engines can process vast amounts of information efficiently, leading to more accurate and timely search results.
What are the Real-World Applications of AI Embedding?
It finds practical use in various real-world applications, particularly in the development of virtual assistants or co-pilots. These co-pilots, powered by AI embedding and Large Language Models (LLMs), enable more advanced natural language interactions, creating more natural and hence enhanced user experiences. They play a vital role in conversational search, understanding user queries, and providing relevant information in a more conversational manner. With AI embedding, virtual assistants can better comprehend user intent, resulting in more accurate, context-aware responses.
AI Embedding Excels in Conversational Search
Embedding and LLMs have enabled conversational search, before these technology realistic conversations and nearest matching where simply not possible. Tools like ConnectingYouNow are now able to interact with users in their own language and hold a conversation that dynamically understands the users intent before displaying only the very best matches.
Here are a few key highlights of how conversational search is a game changer:
Natural language interactions: Enables virtual assistants to understand and respond to user queries more naturally, creating a more human-like conversation experience.
Context awareness: Allows virtual assistants to understand the context of previous user interactions, enabling more relevant and contextual responses.
Improved user experience: Virtual assistants can provide more accurate, real-time information, enhancing the user experience.
Smarter recommendations: Enables virtual assistants to analyse user preferences and behaviour, offering personalised and tailored recommendations.
The Future of AI Embedding
This technology holds promising possibilities, particularly in search. Combining Large Language Models and Embedding allows more content to be understood with less effort, expanding the quantity of content that can be searched which further improves the accuracy and relevance of results. The algorithms to identify the nearest and hence best results are constantly evolving, opening up new possibilities, improving speed and relevance.
Retrieval-augmented Generation (RAG) is rapidly becoming an essential tool for AI Search, repeatedly searching embedded data to help steer search conversations to ensure qualifying questions are relevant which in-turn increases the accuracy of results.
Embedding: Data Privacy and Security
These algorithms are uni-directional. You can create a vector from content, but you can't re-create the content from the vector. Hence each single piece of content is safe.
As with all technology, that is not the full story. To return the actual content it must be possible to link the vector back to the original content. Additionally, the ability to identify content that is similar to other content can provide indirect routes to identify individuals as well as raising ethical concerns. With these risks data privacy and security considerations remain equally as important when Embedding is being utilised.
Can and Should AI Embedding Completely Replace Keyword-based Search?
It has the potential to fundamentally transform search mechanisms, but can it completely replace keyword-based search? The incorporation of AI search is expected to lead to a significant shift from keyword-based search to more natural language interactions. As Embedding continues to advance, search engines will become more adept at understanding user intent, context, and natural language queries, reducing the reliance on specific keywords. While keyword-based search may still have its use cases, the integration of AI embedding in search mechanisms will redefine user experience and provide more accurate, relevant, and personalised search results. This technology paves the way for a more intelligent, user-centric, and seamless search experience.
Conclusion
In conclusion, Artificial Intelligence Embedding has revolutionised the way search engines understand and interpret user queries. It has shifted the focus from keyword-based search to a more nuanced understanding of context and intent. Search engines are able to provide more accurate and relevant results, improving the user experience. However, it is important to note that embedding is not meant to completely replace keyword-based search. Instead, it complements it by adding an extra layer of sophistication. As we move forward, we can expect this field to continue advancing and shaping the future of search technology. It is an exciting time for Search as AI embedding's potential for further innovation is endless.
ความคิดเห็น