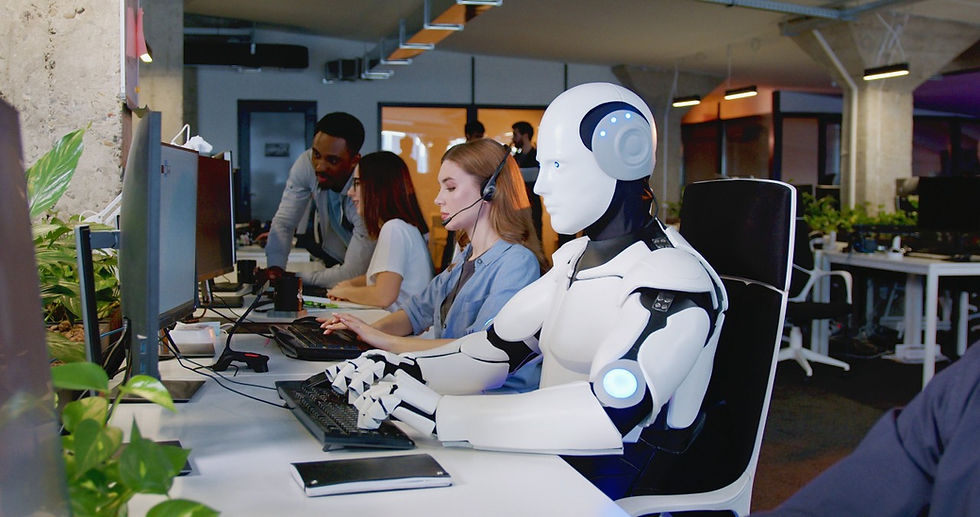
Key Highlights
Analysing feedback data is the first step in identifying recurring support issues and their impact on customer satisfaction.
Common patterns in support requests can be identified through the use of analytics tools and techniques such as ConnectingYouNow's AI Tool - CYN PatternWise.
Leveraging AI for root cause analysis can provide actionable insights for resolving recurring support issues, improving customer satisfaction and reducing costs.
AI-driven solutions, such as automating responses to common queries and implementing predictive analytics, can help reduce support issues.
Integration of AI automation with existing support systems and continuous monitoring and evaluation are key strategies for reducing recurring support issues.
Training staff and customers on AI support features and overcoming challenges in AI automation implementation are important for successful reduction of recurring support issues.
Introduction
Customer support is a crucial aspect of any business, and ensuring customer satisfaction is essential for long-term success. However, many businesses face the challenge of recurring support issues, which can impact customer satisfaction and lead to a decrease in customer loyalty. These recurring issues not only consume valuable time and resources but also hinder the overall customer experience. To address this challenge, businesses are turning to AI automation strategies to identify and resolve recurring support issues effectively.
AI automation leverages the power of artificial intelligence and machine learning to analyse and understand customer support data, identify patterns, and provide actionable insights for resolving recurring issues. By automating responses to common queries, implementing predictive analytics for proactive support, and integrating AI automation with existing support systems, businesses can streamline their support processes and reduce the occurrence of recurring issues.
In this blog, we will explore effective AI automation strategies for reducing recurring support issues. We will discuss the steps involved in identifying common patterns in support requests, the impact of recurring issues on customer satisfaction, and the use of AI tools for root cause analysis. We will also examine case studies and success stories in identifying root causes, as well as best practices for designing AI-driven solutions for recurring issues. Furthermore, we will explore the integration of AI automation with existing support systems, monitoring and evaluating the impact of AI automation, and training staff and customers on AI support features. Finally, we will discuss the challenges in AI automation implementation and the future trends in AI and customer support.
Understanding Recurring Support Issues
Recurring support issues refer to problems or challenges that customers repeatedly face when seeking customer service or support. These issues can range from technical difficulties with products or services to challenges in the user experience or the support process itself. Resolving these recurring support issues is essential for improving customer satisfaction and ensuring a positive customer experience.
Identifying Common Patterns in Support Requests
Identifying common patterns in support requests is a crucial step in understanding and resolving recurring support issues. By analysing support data, businesses can gain insights into the most frequent and common issues faced by customers. This analysis can be done using analytics tools and techniques such as Pareto charts, which help visualise the frequency and impact of different support issues. Some key steps in identifying common patterns in support requests include:
Collecting and analysing feedback data from various sources such as surveys, ratings, reviews, social media, and emails
Using analytics tools to identify the most common support issues and their impact on customer satisfaction
Creating a Pareto chart to prioritise the most critical and frequent support issues
Collaborating with support teams and stakeholders to gain insights and feedback on recurring support issues
By identifying common patterns in support requests, businesses can focus their efforts on resolving these issues and improving customer satisfaction.
The Impact of Recurring Issues on Customer Satisfaction
Recurring support issues can have a significant impact on customer satisfaction and the overall user experience. When customers repeatedly face the same problems or challenges, it can lead to frustration, dissatisfaction, and a decrease in their perception of the quality of service provided. This, in turn, can result in negative reviews, churn, and a decline in customer loyalty.
Moreover, recurring support issues can also put a strain on the service desk or support team as they have to deal with the same issues repeatedly. This can lead to decreased efficiency, increased response times, a higher workload for the support team, and team retention issues.
To ensure customer satisfaction and improve the overall user experience, businesses need to proactively identify and resolve recurring support issues. By leveraging AI automation strategies, businesses can streamline their support processes, identify root causes of recurring issues, and implement effective solutions to address these challenges.
Leveraging AI for Root Cause Analysis
Leveraging AI for root cause analysis is a powerful strategy for identifying and resolving recurring support issues. AI tools can analyse large volumes of support data and identify patterns, trends, and correlations that may not be readily apparent to humans. By applying machine learning algorithms, AI tools can provide actionable insights and recommendations for resolving root causes of recurring support issues. This enables businesses to address these issues at their source and prevent them from occurring in the future.
AI Tools and Techniques for Deep Dive Analyses
AI tools and techniques can enable businesses to conduct deep dive analyses into the root causes of recurring support issues. These tools can analyse support data, customer feedback, and other relevant sources of information to identify patterns and correlations. By using advanced analytics algorithms, businesses can gain a deeper understanding of the underlying factors and conditions contributing to these issues. This allows for more targeted and effective solutions to be implemented, reducing the occurrence of recurring support issues.
CYN Nexus Enterprise AI Hub combined with CYN PatternWise for deep dive analyses include natural language processing (NLP) algorithms for analysing customer feedback, machine learning algorithms for clustering and classifying support data, and predictive analytics models for identifying potential root causes.
Case Studies: Success Stories in Identifying Root Causes
These studies demonstrate how businesses have successfully utilised AI tools and techniques to analyse support data and gain insights into the underlying factors contributing to these issues.
Case Study | Key Findings |
Company XYZ | By analysing customer feedback using NLP algorithms, CYN PatternWise identified a recurring issue with their product's user interface. This led to a redesign of the interface, resulting in a significant reduction in support requests related to usability issues. |
Company ABC | Through deep dive analyses using machine learning algorithms, CYN Nexus discovered a common pattern in support requests related to a specific feature of their software. By addressing the root cause of the issue, they were able to improve customer satisfaction and reduce support tickets by 40%. |
These case studies demonstrate the effectiveness of CYN's AI automation in identifying and resolving root causes of recurring support issues, leading to improved customer satisfaction and a more efficient support process.
Designing AI-Driven Solutions for Recurring Issues
Designing AI-driven solutions is a key strategy for effectively addressing recurring support issues. By leveraging the power of AI automation, businesses can automate responses to common queries, implement predictive analytics for proactive support, and streamline their support processes. These AI-driven solutions can significantly reduce the occurrence of recurring issues, improve response times, and enhance the overall customer experience.
Automating Responses to Common Queries
Automating responses to common queries is an effective way to reduce the occurrence of recurring support issues. By using AI automation, businesses can leverage CYN Access that can provide immediate and accurate responses to frequently asked questions. Some key benefits of automating responses to common queries include:
Improved response times: AI-powered chatbots can provide instant responses to customer queries, reducing the need for manual intervention and improving response times.
Consistency: Automated responses ensure that customers receive consistent and accurate information, reducing the risk of misinformation or confusion.
24/7 support: CYN Access can provide support round the clock, ensuring that customers can access assistance at any time.
Knowledge base integration: AI automation can be integrated with multiple knowledge bases, allowing CYN Access to provide a single view of multiple repositories.
By automating responses to common queries, businesses can free up support agents' time to focus on more complex issues, improving overall efficiency and customer satisfaction.
Implementing Predictive Analytics for Proactive Support
Implementing predictive analytics for proactive support is another effective strategy for reducing recurring support issues. By analysing historical support data, CYN PatternWise can identify patterns and trends that may indicate potential issues before they arise. This allows businesses to take proactive measures to address these issues, preventing them from becoming recurring problems. Some benefits of implementing predictive analytics for proactive support include:
Early detection of potential issues: By analysing historical data, businesses can identify patterns that may indicate potential support issues. This allows them to take proactive action and prevent these issues from occurring.
Improved customer experience: Proactive support can enhance the customer experience by resolving potential issues before they impact the customer.
Resource optimisation: By addressing potential issues before they become recurring, businesses can allocate their resources more effectively and efficiently.
Implementing predictive analytics for proactive support can significantly reduce the occurrence of recurring support issues and improve customer satisfaction.
Integration of AI Automation with Existing Support Systems
Integrating AI automation with existing support systems is essential for ensuring a seamless workflow and maximising the benefits of AI-driven solutions. By integrating CYN's AI tools and techniques with existing support systems such as CRM platforms, businesses can streamline their support processes, enhance data sharing and collaboration among support teams, and improve overall efficiency. This integration enables businesses to leverage the power of AI automation while maintaining continuity and consistency in their support operations.
Ensuring Seamless Workflow with Support Teams
Ensuring a seamless workflow with support teams is crucial for the successful integration of AI automation with existing support systems. By providing support teams with the necessary training and resources, businesses can ensure that they can effectively utilise CYN AI tools and techniques in their daily operations. Some key considerations for ensuring a seamless workflow with support teams include:
Training: Providing comprehensive training on AI tools and techniques, as well as the integration with existing support systems, is essential for support teams to effectively utilise these technologies.
Collaboration: Facilitating collaboration and knowledge sharing among project and support teams is vital for maximising the benefits of AI automation. This can be achieved through regular team meetings, cross-training, and the use of CYN Access search interface.
By ensuring a seamless workflow with support teams, businesses can maximise the impact of AI automation on reducing recurring support issues.
Best Practices for Integrating AI Tools
Integrating AI tools into existing support systems requires careful planning and consideration. To ensure a successful integration, businesses should follow best practices such as:
Analysing existing workflows and processes: Before integrating AI tools, businesses should analyse their existing workflows and processes to identify areas that can benefit from automation and AI-driven solutions.
Customising AI tools: AI tools should be customised to align with the specific needs and requirements of the business. This may include training the AI algorithms to understand the business context and terminology or customising the user interface of AI tools to match the existing support systems.
Testing and monitoring: Regular testing and monitoring of the integrated AI tools are essential to ensure their effectiveness and accuracy. This includes analysing the performance of AI algorithms, monitoring customer feedback, and making necessary adjustments or improvements. This is provided within the CYN platform - CYN TrustForge
By following these best practices, businesses can successfully integrate AI tools into their existing support systems and maximise the benefits of AI automation in reducing recurring support issues.
Monitoring and Evaluating AI Automation Impact
Monitoring and evaluating the impact of AI automation is crucial for assessing its effectiveness in reducing recurring support issues. By tracking key performance indicators (KPIs) and conducting regular audits, businesses can measure the impact of AI automation on customer satisfaction, support ticket resolution time, and overall support team efficiency. This monitoring and evaluation process enables businesses to identify areas for improvement and make necessary adjustments to their AI automation strategies.
Key Performance Indicators to Watch
When monitoring the impact of AI automation, businesses should track key performance indicators (KPIs) to assess its effectiveness in reducing recurring support issues. Some key KPIs to watch include:
Customer satisfaction score: Measuring customer satisfaction through surveys or feedback ratings can provide insights into the impact of AI automation on overall customer satisfaction.
Support ticket resolution time: Tracking the time it takes to resolve support tickets can help businesses assess the efficiency of AI automation in resolving recurring support issues.
Support team efficiency metrics: Monitoring metrics such as average handle time, first call resolution rate, and ticket backlog can provide insights into the impact of AI automation on support team efficiency.
Audits and reviews: Regular audits and reviews of AI automation processes and outcomes can help identify areas for improvement and ensure the accuracy and effectiveness of AI-driven solutions.
By monitoring these key performance indicators and conducting regular audits, businesses can evaluate the impact of AI automation on reducing recurring support issues and make necessary adjustments to their strategies.
Continuous Improvement Based on AI Insights
Continuous improvement based on AI insights from CYN PatternWise is essential for optimising the effectiveness of AI automation in reducing recurring support issues. By analysing the insights businesses can identify areas for improvement, refine their AI-driven solutions, address product testing challenges, and iterate on their support processes. Some key strategies for continuous improvement based on AI insights include:
Regular feedback loops: Establishing regular feedback loops with support teams and customers can provide valuable insights and suggestions for improving products.
Actionable insights: AI tools should provide actionable insights that can be used to drive meaningful improvements in support processes and the products being supported. These insights should be specific, relevant, and actionable.
Iterative approach: Adopting an iterative approach to AI automation allows businesses to continuously refine and improve their AI-driven solutions based on real-time data and feedback.
By embracing continuous improvement based on AI insights, businesses can maximise the effectiveness of AI automation in reducing recurring support issues and improving overall customer satisfaction.
Training Staff and Customers on AI Support Features
Training staff to utilise AI self-service support features is crucial for maximising the benefits of AI automation and ensuring a positive user experience. Furthermore, educating customers on using CYN's intuitive Access support features can help them make the most of the available resources and enhance their overall experience.
Educating Customers on Using AI-Enhanced Support
Technology leaders can provide resources such as user guides, tutorials, and FAQs through CYN Access. Ensuring these resources are easy find will help customers navigate and make the most of AI support features. Technology leaders can empower business partners to find solutions to their problems more efficiently, reduce support ticket volume, and improve overall satisfaction.
Overcoming Challenges in AI Automation Implementation
Implementing AI automation can come with its fair share of challenges. However, overcoming these challenges is crucial for successfully reducing recurring support issues. Some common challenges in AI automation implementation include privacy and security concerns, managing customer expectations and experience, and ensuring a smooth integration with existing support systems. By addressing these challenges through CYN's careful planning, training, and collaboration, businesses can successfully implement AI automation and effectively reduce recurring support issues.
Managing Internal Customer Expectations and Experience
Managing customer expectations and experience is crucial for successful AI automation implementation. Technology leaders should set clear expectations about the capabilities and limitations of AI-driven support and ensure that internal customers understand how to effectively utilise AI-enhanced features.
Future Trends in AI and Customer Support
The future of AI and customer support is promising, with several emerging trends set to revolutionise the industry. These trends include the adoption of emerging technologies such as natural language processing, chatbots, and virtual assistants to enhance customer interactions and support experiences. Additionally, advancements in predictive analytics and machine learning algorithms will enable businesses to leverage AI automation for more intelligent and proactive support. As AI continues to evolve, businesses should stay informed about these future trends and adapt their strategies accordingly to stay ahead of the curve and effectively reduce recurring support issues.
Preparing for the Next Wave of AI Innovations
Preparing for the next wave of AI innovations is crucial for businesses to stay competitive and effectively reduce recurring support issues. This requires collaboration and alignment among stakeholders, including support teams, IT departments, and leadership. By staying informed about AI advancements and actively participating in industry discussions and events, businesses can prepare for the future and ensure that their AI automation strategies are optimised to address recurring support issues.
Conclusion
In conclusion, effective AI automation strategies hold the key to reducing recurring support issues and enhancing customer satisfaction. By leveraging CYN for root cause analysis, designing AI-driven solutions, integrating with existing support systems, and monitoring impact, businesses can streamline support processes and improve overall efficiency. Embracing future trends in AI and continuous improvement based on insights will further revolutionise customer support experiences.
Frequently Asked Questions
How Does CYN's AI Identify Recurring Support Issues?
CYN PatternWise identifies recurring support issues through the analysis of support data using advanced analytics techniques. By identifying patterns and trends in support requests, AI algorithms can recognise recurring issues and provide insights for their resolution.
Can AI Automation Completely Replace Human Support Agents?
While AI automation can greatly enhance customer support processes, it cannot completely replace human support agents. Human support agents bring a level of empathy and understanding that AI algorithms cannot replicate. However, AI automation can assist human support agents by automating repetitive tasks, providing data-driven insights, and improving overall efficiency.
Comments