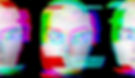
Artificial intelligence (AI) has made significant advancements in recent years, transforming the way we interact with technology and the world around us. From co-pilots to language models, AI has the ability to generate content that mimics human-like responses. However, with this power comes the potential for AI hallucinations, where the generated content may not always be accurate or reliable. In this blog, we will explore the causes of AI hallucination and discuss possible solutions to address this growing concern.
Understanding AI Hallucinations
To understand AI hallucination, it is essential to first grasp the concept of artificial intelligence itself. AI refers to the development of computer systems that can perform tasks that typically require human intelligence, such as understanding natural language, recognising images, or making decisions. AI hallucination, on the other hand, is a phenomenon where the generated content produced by Artificial Intelligence systems deviates from reality or exhibits false information.
At a recent conference, a representative of a vendor put it very well, "Generative Artificial Intelligence has a fluid relationship with the truth". Given the underlying large language models work on probabilities, the generated responses aren't tied to the actual truth, only a probably truth.
What are AI Hallucinations
AI hallucination can occur in various forms, stemming from generative AI models and their underlying large language models (LLMs). These models, powered by neural networks, have the capability to generate content based on training data. This generative process, however, can lead to hallucinations when the generated content deviates from reality, providing incorrect or misleading information.
Why Should we be Concerned about Them
The growing presence of Large Language Models, such as OpenAI's ChatGPT and Google's BARD, has highlighted the prevalence of hallucination. These models are trained using vast amounts of data, including news articles, social media posts, and internet content. While this training data provides a wealth of information, it can also introduce biases, misinformation, and factual errors, which may manifest as hallucinations in the generated content.
AI hallucination not only poses a challenge to the accuracy of Artificial Intelligence systems but also raises concerns about user trust. When AI systems provide incorrect information or spread false content, it can erode the trust users place in these technologies. Hallucinations are rapidly becoming a major part of a growing list of ethical concerns related to AI.
How do AI Hallucinations Occur?
AI hallucinations occur as a result of the training process, the underlying mechanisms of generative models and the desire of the tools built on them to answer, apparently authoritatively, every question and request posed to them.
These models, powered by neural networks, are trained to analyse patterns in training data and generate content based on those patterns. However, the training data itself plays a crucial role in determining the outcomes of the generative process.
During the training phase, the neural networks learn from the training data, capturing the statistical relationships and patterns present within the dataset. As a result, the generative model becomes a reflection of the data it was trained on, inheriting biases, factual errors, and inconsistencies present in the training data. This can lead to the generation of hallucinated content that deviates from reality.
A factor that is equally responsible for hallucination is generative AI's built in desire to please! It really doesn't like saying that it can't answer a question, and given that it currently can't differentiate between truth and fiction, it will happily make up a response, and not let the user know any level of trust that should be applied. I have yet to have seen any generative model respond with real life expression, "I am not 100% sure of this one, but if I had to guess...".
To mitigate the occurrence of hallucination, it is crucial to have a deep understanding of the underlying reality the generative models aim to capture. By comprehending the nuances of real-life scenarios, the models can better distinguish between factual information and hallucinated content. This understanding can be achieved through the careful curation of training data, the implementation of process supervision, and the utilisation of algorithms that prioritise the generation of accurate content.
OpenAI's ChatGPT, Google's Bard and the Rest: All display Various Types of Hallucinations
AI Hallucination can take on various forms depending on the type of model and the task it performs. In the realm of natural language generation, hallucinations often manifest as factual errors or the inclusion of incorrect information in the generated content. Language models, trained on a vast corpus of text, have the potential to confabulate information or produce responses that align with the training data but do not reflect reality.
In the field of computer vision, hallucination can occur when a model misclassifies or misrepresents visual input. This can lead to false interpretations of images, videos, or other visual data, potentially causing misinformation or inaccurate analysis. Adversarial attacks, where purposely crafted inputs manipulate the model's output, further exacerbate the occurrence of hallucination in computer vision systems.
The different forms of hallucination highlight the challenges and complexities involved in training AI models. As advances in machine learning continue, addressing these hallucination types becomes critical to enhance the reliability and trustworthiness of AI-generated content.
Preventive Measures against AI Hallucinations
Preventing AI hallucinations is a multi-faceted task that involves various preventive measures. By leveraging machine learning techniques, dataset curation, and algorithmic improvements, the likelihood of hallucination occurrences can be minimised. These measures aim to ground the Artificial Intelligence models with relevant data, enhance the understanding of the underlying reality, and reduce biases, factual errors, and confabulation. In addition techniques are emerging to add safety nets to the content generated from the models to further anchor them in reality.
Model Training: Grounding AI Models with Relevant Data
To reduce the occurrence of AI hallucination, grounding AI models with relevant data is of utmost importance. Relevant data provides the necessary context and factual information for the models to generate more accurate content. Several techniques, such as process supervision, can be employed to guide the generative models and ensure the content aligns with the intended reality.
Process supervision involves incorporating additional context or guidance into the training process, helping the model produce more reliable and grounded outputs. By iteratively training the model with feedback from human reviewers, the model learns to separate correct information from hallucinated content. This iterative process helps refine the model's understanding of reality and improves the quality of the generated content.
Grounding AI models with relevant data not only reduces the likelihood of hallucination but also addresses ethical concerns in AI. As Artificial Intelligence technologies become more prevalent and influential, ensuring the generation of accurate and trustworthy content becomes crucial for user trust and the responsible deployment of AI systems.
Including a diverse range of training data is essential to mitigate biases and ensure a comprehensive understanding of real-world scenarios.
Diverse datasets help models generalise better, reducing the likelihood of hallucinations and ensures that minority groups and views are suitably represented.
A variety of factors, perspectives, and contexts captured in a diverse dataset can enhance the model's understanding of the underlying reality.
By training models on diverse data, the generation of hallucinated content can be reduced, enhancing the accuracy of the generated content.
Retrieval Augmented Generation (RAG): The Hidden Weapon Against Hallucination
LLMs continue to improve as do other AI models, they are trained on ever improving data sets and their probability models become more and more effective, but having a more traditional algorithmic safety-net is likely always to be encouraged. At present, the emerging solution in this area is Retrieval Augmented Generation (RAG).
RAG enhances content generation by integrating information retrieval during the process. It retrieves relevant information from a large knowledge source, like a database or the web, to inform the generation of content. This approach improves content coherence, factual accuracy, and diversity in generated content by effectively checking the generated content against an external, trusted knowledge base before presenting the result.
Products like the search co-pilot, ConnectingYouNow, use the information in their potential search results to validate results and also to influence the content produced by the Large Language Model, this is proving incredibly effective in ensuring the correct answer is given in the vast majority of cases.
RAG can be seen as adding the element of truth missing from the base Large Language Models, increasing reliability and hence trust in the generated responses.
Can AI Hallucinations be Fully Eliminated, or Just Minimised?
The generative nature of AI models, coupled with the complexities of human psychology, leaves room for the potential generation of hallucinated content. Achieving a perfect understanding of the underlying reality across all possible outcomes is a daunting task, making the complete elimination of hallucinations a difficult goal to achieve. This complexity is driving the establishment of preventative approaches such as RAG and justifies their continuing use in trusted tools.
Conclusion
In conclusion, AI hallucinations are a phenomenon that occurs when artificial intelligence systems generate outputs that are not based on actual data or reality. This can lead to incorrect or misleading information being produced. While it is challenging to completely eliminate hallucinations, there are preventive measures that can be taken to minimise their occurrence. These include using diverse and representative data during the training of models, grounding the models with relevant and accurate information and including prevented techniques like Retrieval Augmented Generate. By implementing these measures, we can work towards improving the reliability and trustworthiness of Artificial Intelligence systems. It is important to continue researching and developing solutions to address AI hallucinations and ensure the responsible and ethical use of artificial intelligence.